What is Artificial Intelligence?
Artificial Intelligence is an extremely broad term which involves the use of computational tools & algorithms in order to complete tasks, which in the past required human level sophistication. In other words computer systems are being designed to mimic human intelligence.
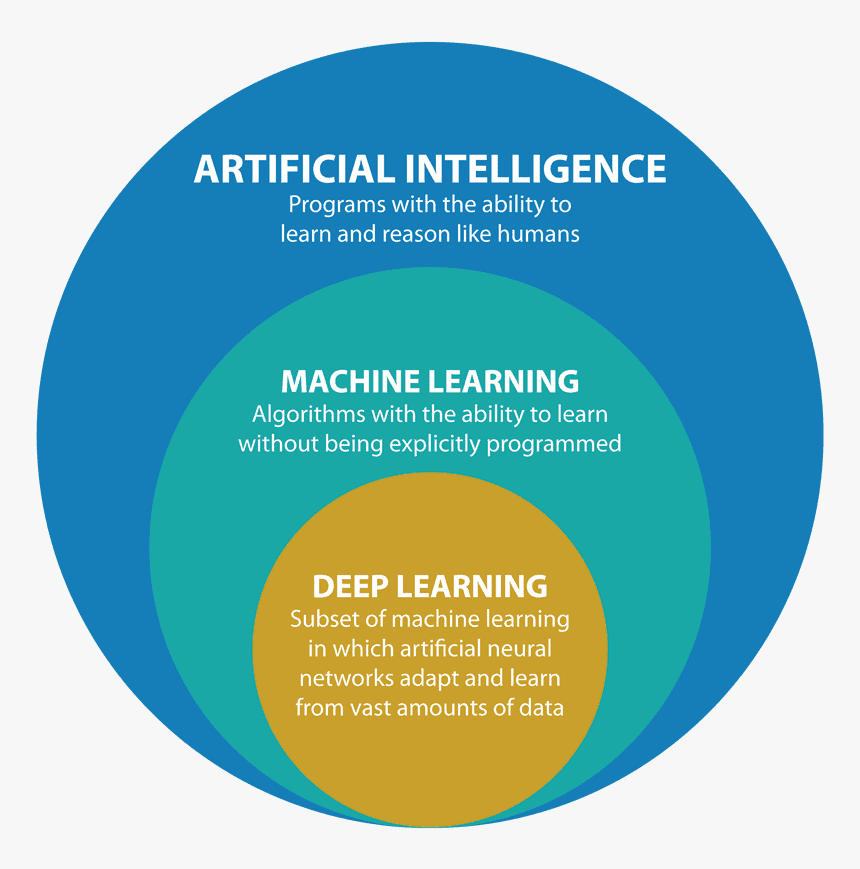
Artificial Intelligence Machine Learning Source: Argility
Evolution of Artificial Intelligence
Artificial intelligence (AI) is a term which was developed from classical philosophers attempting to explain human thinking as a symbolic system. Then in 1956, Dartmouth College officially coined the term “Artificial Intelligence” at a conference.
Since then Artificial Intelligence generally took a sleepy 25 years where researchers ruminated & computing technology gradually developed & costs declined, thanks to Moore’s Law & the 7 stages of AI.
Today AI has exploded in popularity, according to International Data Corporation (IDC) global spending on AI will jump from $85.3 billion in 2021 to over $204 billion by 2025! That is a compounded annual growth rate (CAGR) of a massive 24.5%, making it one of the fastest growing technology fields in terms of investment.
The global pandemic has served as a catalyst which has accelerated existing trends in the technology. Companies have reassessed there technology stack & began to use AI as a tool or service to improve back office systems & customer facing user interfaces.
According to a 2021 survey by Mckinsey, 56 percent of all respondents reported AI adoption in at least one business function, the results also suggest emerging market economies such as India, China, the middle east & north Africa report increased adoption acceleration up 57 percent in 2021, from just 45 percent in 2020.
Impact on the Bottom Line
The cost savings & revenue increases from AI has resulted in at least 27% of the companies surveyed by Mckinsey reported at least 5 percent of their earnings before interest and taxes (EBIT) attributable to AI systems.
What is Fintech?
Fintech is the use of technology in financial applications. Finance has had a symbiotic relationship with technology for many years from online banking which started over 20 years ago to todays “Insurtech” companies.
The finance industry intermediates money & risk, transferring it from those who don’t want it, to those that can manage it profitably such as insurance, banking etc.
Financial Firms sit at the neck of an hour glass and thus are in a lucrative position to collect economic rents, the use of technology & in this case AI allows this to be done more accurately, efficiently and at a lower cost.
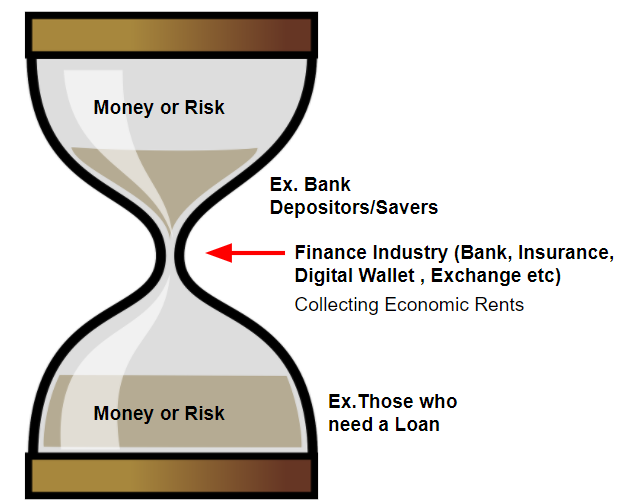
Finance industry sits at the neck of an hour glass. Image Created by Author Ben Alaimo at www.Motivation2Invest.com
AI Applications
AI has a broad spectrum of applications, these are in areas where computer automation technology can do a better, faster or cheaper job than a human.
According to a 2021 AI Survey by McKinsey, the top three use cases are:
- Service/operations optimization
- AI based enhancement of products
- Contact Center Automation
The largest percentage point increase in the use of AI was for companies to allocate their spending budget on areas such as marketing.
AI Applications in Fintech
1. Personalization
Personalization is the key to profits, from marketing campaigns to custom user experiences. Many smart fintech app’s and wallets are now offering custom/personal consumer experiences. The same way Youtube’s algorithm shows you videos on the home page which “people like you” also watched, the same personalization is occurring in the finance industry.
i. Personalized Expense Tracking
Fintech companies such as Brex offer expense tracking which can used to help small businesses make optimisation decisions much easier, which means no more excel!

BREX expense tracking. Source: www.Brex.com
There are many applications for this for example you can spot a certain expense is growing rapidly and may need to be curbed. As a personal example, I am an investor into a Barbershop business and noticed the PPE expenses had risen massively in 2020 and were now eating heavily into the business profits.
Now although some of these expenses were necessary & vital for the health of customers others weren’t so much. I noticed the business provided free masks to every customer, rather than encouraging the customer to bring their own like many large retailers.
Thus we changed our policy & most people were happy to bring their own masks which helped lower the PPE costs especially as prices of health care related PPE had inflated to extortionate levels.
Many incumbent U.S. banks such as Bank of America, JP Morgan Chase & Wells Fargo, have also launched mobile banking app’s which allow customers to track their expenses, pay bills and communicate with their bank in a frictionless way.
ii. Personalized Financial Advice (Robo Advisors)
Financial Advice is going through a period of democratization, historically a consumer would have to set up a meeting with an expensive financial advisor to discuss their options and then pay large fee’s for the service or indirectly through product recommendations. However, today Robo Advisor services are offer low cost financial advice in a fast, efficient & personalized manner.
iii. Personalized Investing Advice
Taking things one step further from Financial Advice, Robo advising applications can offer investment advice and various portfolio types based upon the persons lifestyle, income & risk tolerance.
In terms of Trading, Bloomberg launched Alpaca Forecast AI Prediction Matrix, an AI powered app which provides short-term market price forecasts for major markets such as USD/JPY, EUR/USD etc.
Robo Advisory Platforms include: Advisory Platforms: Acorns (2014), Plum (2016) Betterment (2008), Blooom (2013), Ellevest (2014), Groww (2013), M1 Finance (2015), Motif (2012),
Nutmeg (2012), Personal Capital (2009), SigFig (2006), SoFi (2011),
Tastyworks, Wealthfront (2008), Wealthsimple (2014)
Major intuitional Investors such as the secretive Renaissance Capital have been using AI models for many years to beat the market consistently.
2. Credit Decisions & Alternative Data
Traditional Credit scoring systems only use tradition data such as past loans and credit cards etc. However, the collection of alternative data such as information about utility payments, rental payments and consumer habits offers a whole new level of service.
This vast data set can be analysed with Artificial Intelligence to create accurate models of the potential borrower and thus offer them competitive & low cost credit decisions.
This can also help to increase Financial Inclusion & avoid bias which may occur in traditionally human managed systems. However, the AI systems must include what is called “Explainability” the ability to explain how the AI model (Black Box) has analysed the data.
Many Digital Banks & lenders have begun to implement AI & Alternative data to make improved credit decisions.
Personalized Loans
Companies such as Square (now Block) (NYSE: SQ) and Brex are offering personalised loans to small businesses from the data collected on company, by tracking their business expenses and finances. This has a multitude of benefits for both the consumer & financier.
For the consumer (small business), this means faster loan approval, better rates & flexible payback periods with revolving credit. For the lender, AI & big data allows lower cost customer service, scalable platforms and lower underwriting risks.
3. Customer Service
As the 2021 McKinsey Survey noted, Contact Centre Automation was a top 3 application of AI in 2021. The use of Cloud Contact centres & smart chats bots are providing faster customer service & lower costs for the businesses incorporating them.
Now although many people who I have asked personally about this say they prefer to speak to someone on the phone there is no doubt that the feasibility of offering physical customer service at scale is almost impossible. For example, Google (Alphabet) is famous for not really having any formal phone customer service. They prefer to setup Forums, support requests etc.
According to a Study by Super Office, the most important attribute of the customer experience to customers is a fast response time (at 75%) above just 37% who opted for a “person to speak with”.
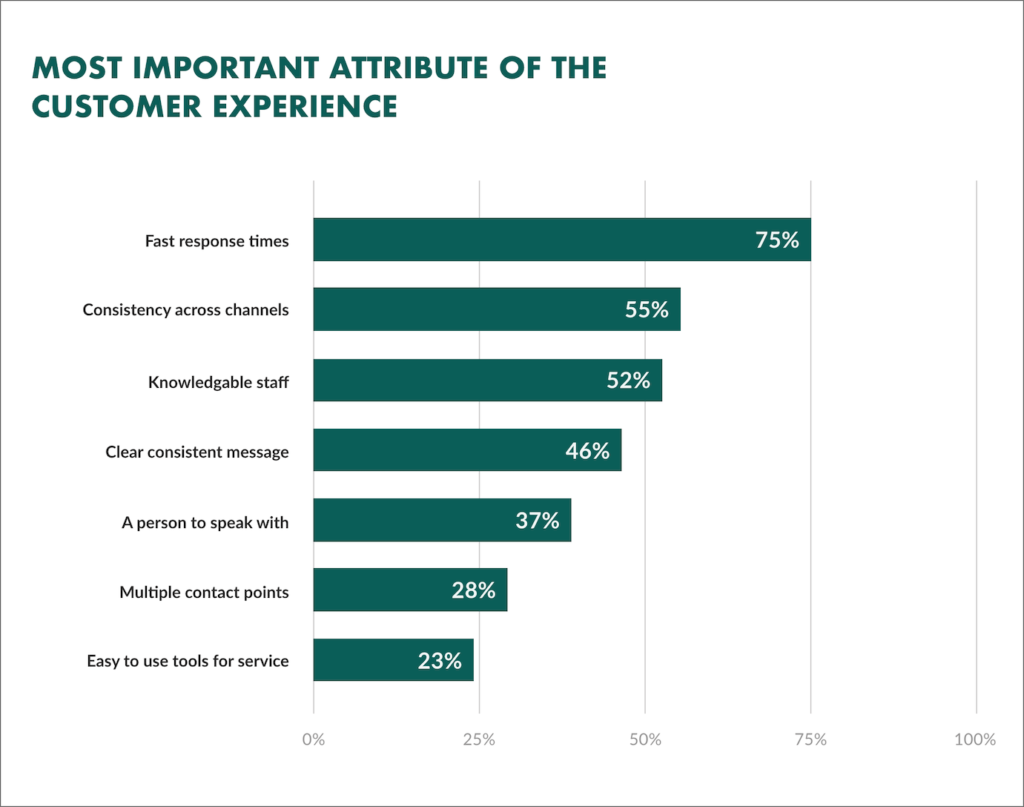
most-important-attribute-customer-experience. www.SuperOffice.com
Human Call Center workers also experience a terrible time in many cases, as they are the first point of contact for angry customers. According to a study by QATC .
The average turnover rate for a call center is 30 to 40%, but some centers see figures as high as 100% in a single year! This is mainly down to the excessive demands & high stress levels of the job. Buzz Feed offering an interesting post which shares “Call Center horror stories“.
I thought it was common knowledge, but apparently it’s not: when a call center puts you on hold, we can still hear you! 😅 Shady sales folks will use what you say on hold against you, stay safe outchea lol.
— The People’s Artist (@Tacocassoart) January 9, 2022
High staff turnover results in higher recruiting & onboarding costs in addition to lower employee morale. There is also the risk that one day, a call center worker may “crack” and lash out back at a customer, this could cause major brand damage especially for high ticket customers.
Thus the use of AI chat bots can solve this issue offering a faster service & saving for the revolving door that is staffing contact centres.
4. Natural Language Processing (NLP)
Natural language processing (NLP) is a branch of artificial intelligence which focuses on helping computers to understand human writing and speech. This is a challenging task as it involves a lot of unstructured, non uniform data.
Natural language processing (NLP) technology has improved massively over the years and the service is set to become more “human like” and accurate. The most common examples include Voice controlled Virtual Assistants such as Amazon Alex, Google Home and Apples Siri.
Amazon Alexa even offers the ability to check balances & make payments. For Example, if you shop with your Alexa-enabled device and Amazon Pay, you don’t have to waste time inputting your payment or shipping information and can pay instantly. For example, you can say “Alexa, Buy two movie tickets”
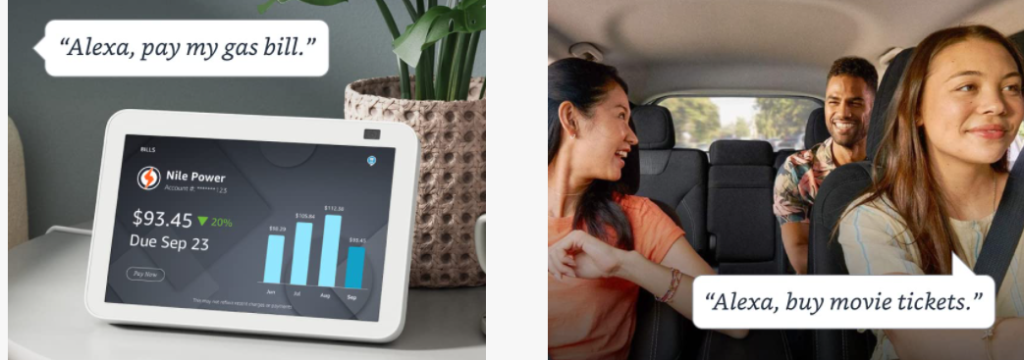
ALEXA AND AMAZON PAY. Source: https://pay.amazon.com/
5. Risk Management
AI allows the analysis of big data to create more accurate models for underwriting. This could be to increase the accuracy of those people who are selected eligible for loans as mentioned with Squares small business loans.
AI can also be used to more accurately analyse financial risk for actuaries. For example, Insurtech company Cytora is said to be building the “Underwriting of the future” through the use of AI and big data. They have developed a “Risk Engine” which ingests data from thousands of sources to obtain a more comprehensive view of the market than any single insurer provides. Read full report here, Cytora Risk Engine.
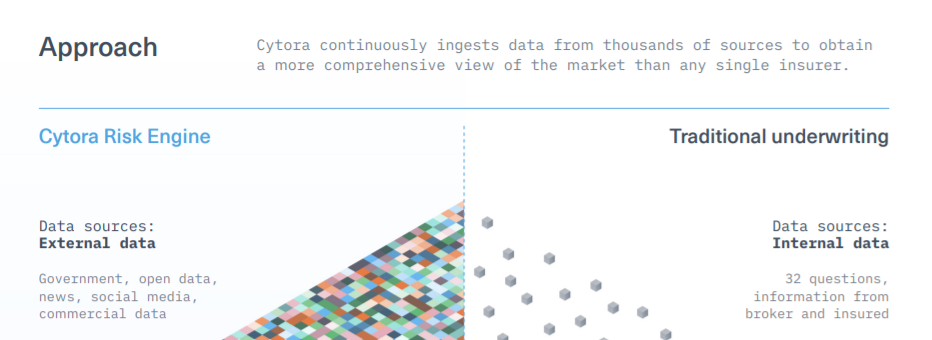
Cytora risk Engine 1. Source: https://cytora.com/wp-FQv6a6pa/wp-content/uploads/2019/01/Cytora_Risk_Engine_Benchmark_Report.pdf
6. Telematics & Insurtech
The use of Telematics to track customers driving and increase the accuracy of risk models is becoming increasingly popular. For example, Metro Mile is company which charges auto insurance based upon how often you drive & where you drive, rather than time of policy. ByMiles in the UK offers a similar service.
Even incumbents such as Metlife are offering an Auto & home usage based insurance program called MyJourney. This uses a smartphone app to monitor customer driving.

METLIFE MY JOURNYE APP. Source: https://appadvice.com/app/metlife-my-journey/1199480580
The app tracks total miles driven, time of day, road type, conditions, hard braking, harsh acceleration and even phone based distraction! The beauty of this system is it doesn’t just improve the accuracy of customer profiles & lower underwriting risk but it also improves the driving of the people using the app.
As the financial incentive may encourage you to drive in less harsh conditions or check your phone less as you know it is impacting your wallet. The system calculates & immediately displays a score for each trip between 1 and 100 with 100 being the safest possible trip.
This adds a gamification to the system which should help people to lower insurance costs, example a consumer my say “last time I scored 65, next time i want to score 80+ and lower my insruance costs.
The backbone of this system is by TrueMotion (acquired by Cambridge Mobile Telematics) , a boston based company which specialises in combining mobile data and Artificial Intelligence to analyse areas of higher traffic accident risk.
There is a great post here on Insurtech and Machine Learning to find out more.
7. Fraud Prevention
Artificial intelligence is great at analysing patterns in data and anomalies in services and thus has been very successful in combating financial fraud.
i. Credit Card Fraud
AI is exceptional at preventing credit card fraud which has risen thanks to the growth in e-commerce. Fraud Identification systems analyze customer behavior, location & purchasing habits to trigger a security alert in the event of irregularities detected against the cost model of each person.
For example, using 6 months worth of data the AI system profiles Sally as living in the USA and spending approximately $100 per day on Coffee at her local Starbucks, Restaurants and at the local shopping mall. But then one day there is a large $1000 purchase reported in Sri Lanka…this would be detected as an anomaly in the system.
Years ago, systems such as these tended to overreact if for example you went on vacation it was usually best to inform your bank prior to avoid having your card blocked. However, these days your smartphone location data with the banking app can be used to detect your location & thus improve consumer experience & reduce false signals.

Cybersecurity & Fintech
ii. Money Laundering
Banks use artificial intelligence to expose and prevent money laundering. The Bank Secrey Act (BSA) is the primary U.S. anti-money laundering (AML) law. To help enforce this AI is used to detect suspicious activity (large deposits, withdrawals or international transactions) and then report this to the relevant authority for further investigation.
Data Aggregators like Floyd works with financial giants such as Goldman Sachs, American Express & Citi to create fraud-detection models. Plaid integrates bank with the client’s app to ensure secure financial transactions.
Conclusion
AI as a tool or service is rapidly changing the business landscape across the financial industry. This is applicable across a vast array of applications on both the consumer facing & back end side of operations. As the technology improves in terms of speed, accuracy & increased data sources, the applications should also improve exponentially.
Technologies such as Blockchain & smart contracts could further expand range of potential applications but also disrupt incumbent finance as it mitigates the need for an intermediary.
The increased popularity of the “Metaverse” and VR should offer a range of new experiences such as self help Virtual reality systems.
Back office systems can be streamlined & cost lowered freeing up human capital to focus more on the complex problem solving which is still better to suited to human judgement….at least for now.